Introduction
This week's lab was yet another continuation of the weeks prior. Last week consisted of testing out the microclimate geodatabase in the field, which then led to the understand of what worked and what did not. For lab this week, a refined geodatabase was created for the entire class (thanks to Zach Hilgendorf) in order to be able to go out into the field to collect data and bring it back into one ArcMap document. The purpose of this lab was to connect the past few week's knowledge and create the full microclimate data set for the entire campus by using the
Trimble Juno GPS (see figure 1) to record the data in combination with the
Kestrel weather meter (see figure 2).
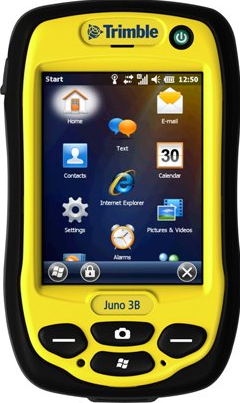 |
Figure 1. This is the Trimble Juno GPS that was used in the data collection during this lab. It has the capabilities of an ArcPad application which allows for data collection directly into the mobile version of ArcMap. |
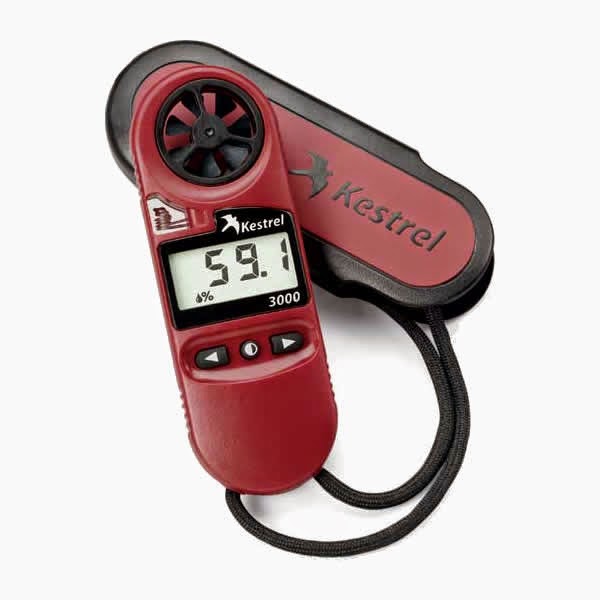 |
Figure 2. This is the Kestrel 3000, which measured temperature (at the surface and 2 meters), wind speed, wind chill, dew point, and percent humidity for the microclimate data collection in this lab. |
Methods
Before heading out into the field, it was vital that everyone was able to deploy the correct map and database onto their Juno to ensure that no mistakes were made. If errors were realized either in the field or once back in the lab, the entire data collection process would have to be redone to ensure accuracy.
The University of Wisconsin- Eau Claire campus is geographically distinctive in that it has a large hill (or more accurately a terrace) that divides lower campus from upper campus as seen in figure 3. Lower and upper are divided by the string of trees in the south west portion of the map. In addition, the Chippewa River runs along the north side of campus with a footbridge running over it for pedestrians to cross to get off campus or to the two academic buildings over the river.
For the data collection, the same features were collected as for the last data collection: temperature at the surface, temperature at two meters, windchill, dewpoint, humidity (%), wind speed, ground cover, and notes. One feature was neglected, wind direction, because of lack of compasses for the class. The class was instructed to collected about 100 GPS points per groups of two and assigned a specific area on upper or lower campus to cover. One member of the group was in charge of using the Kestrel to determine the various climatic readings, while the other member inputted the data taken from the Kestrel to the Trimble Juno.
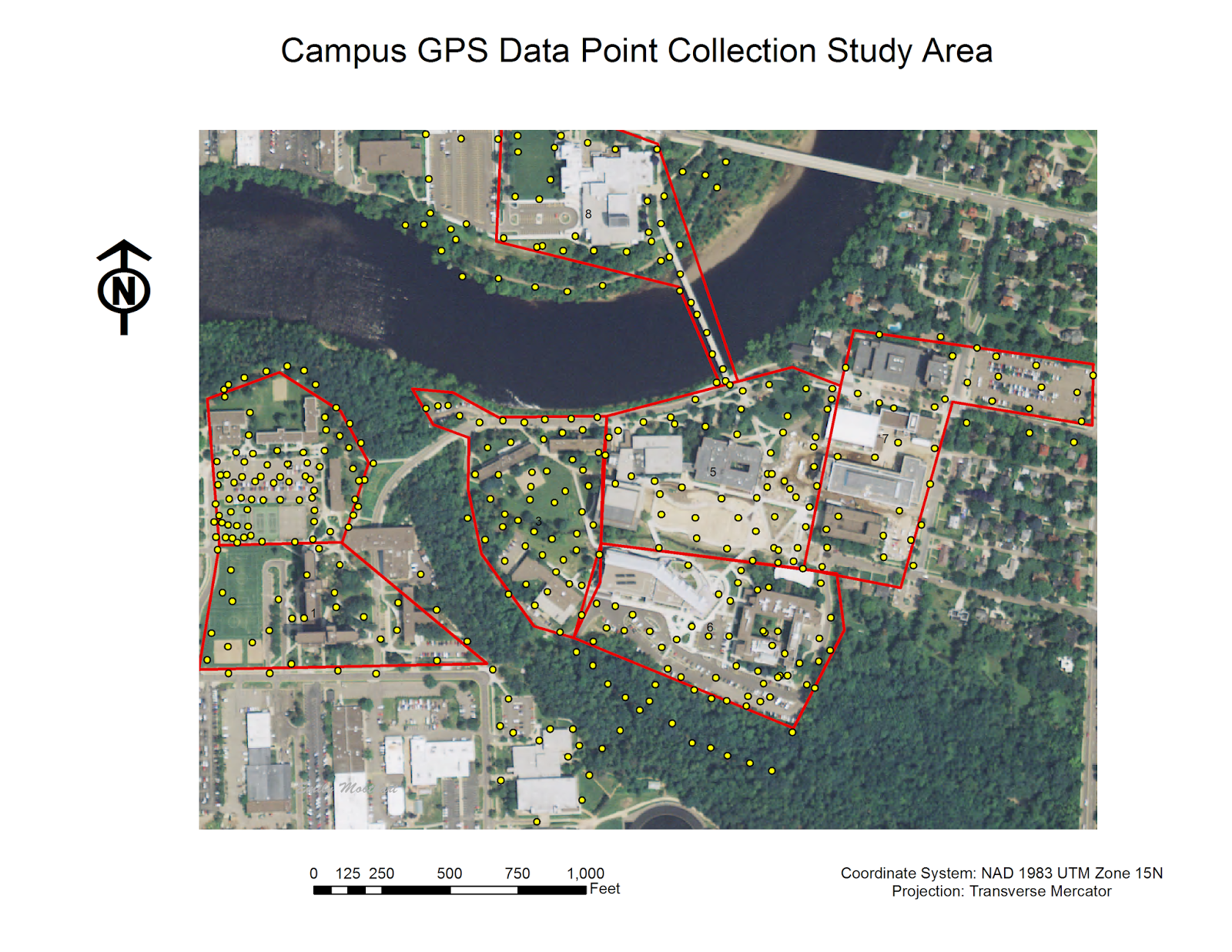 |
Figure 3. Campus GPS Data Point Collection Study Area. This map depicts all of the points that were collected throughout lower campus (central portion of map) and upper campus (western portion of map). The red polygons indicate the data collection areas assigned to groups of two. |
Once all of the data was collected by the class, the data was imported into a single feature class in one ArcMap file. This allowed the entire class to have access to each other's points in an editable form. In order to see continuous microclimate data for the campus, an inverse distance weighted (IDW) interpolation was performed. This interpolation technique was used for its ability to see local variation with a large number of sampling points.
Overall, the data showed a clear indication that Putnam Park (the heavily wooded region in the south west portion of the map) was cooler in temperature than the rest of the campus.
One weather measurement that was run was dew point (see figure 4). This is defined as the temperature at which the moisture in the air forms visible drop of water. This test was conducted in Fahrenheit.
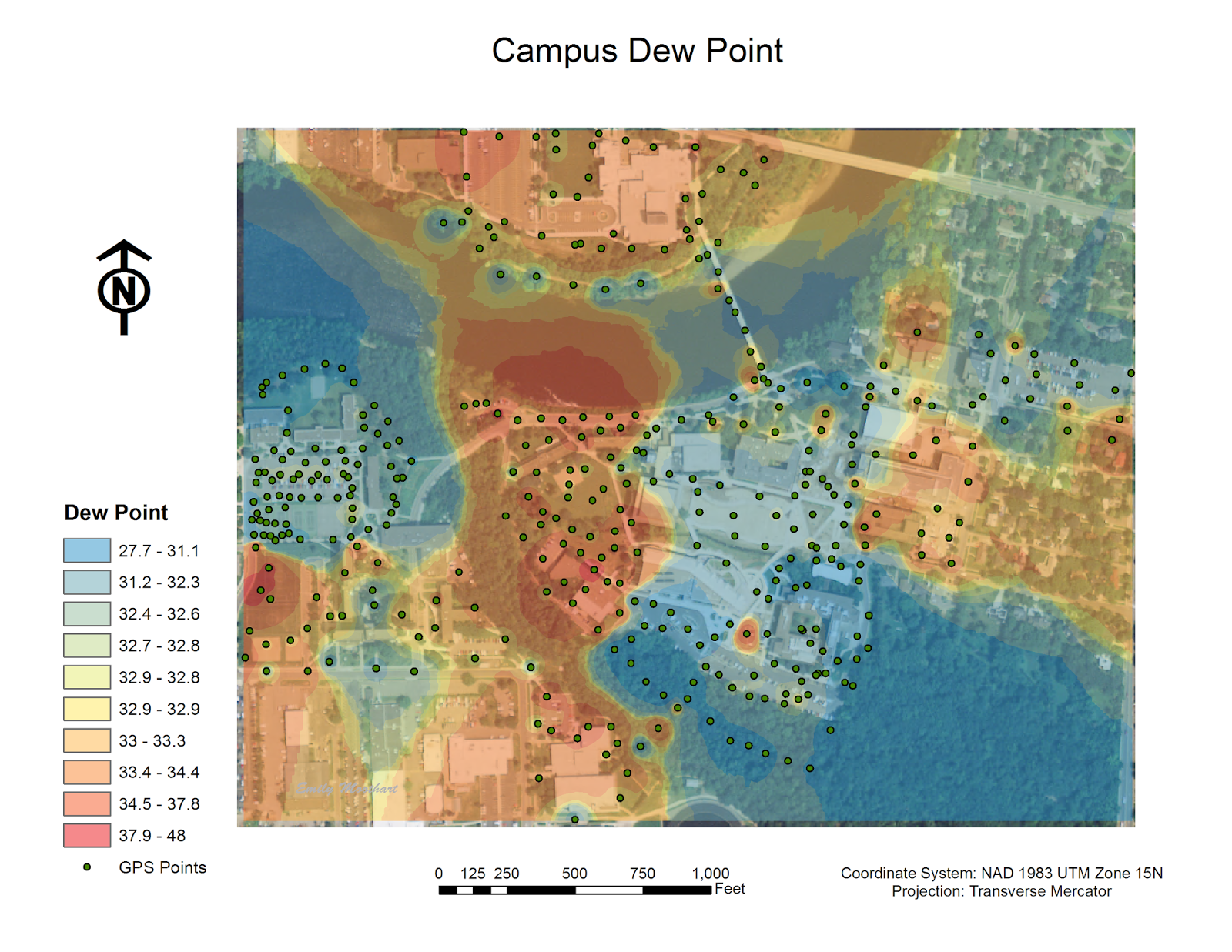 |
Figure 4. Campus Dew Point (Fahrenheit). The regions that have higher dew point are generally areas around open water, or in the case of this particular day on campus, waterlogged because of the snow melting. The lowest dew point is located in the general area of the parking lots which contain a lot of cement, and likewise almost no water. |
Relative humidity is another variable that was tested for the campus microclimate (see figure 5). It is defined as the ratio of partial pressure of water vapor to the equilibrium vapor pressure of water at the same temperature.
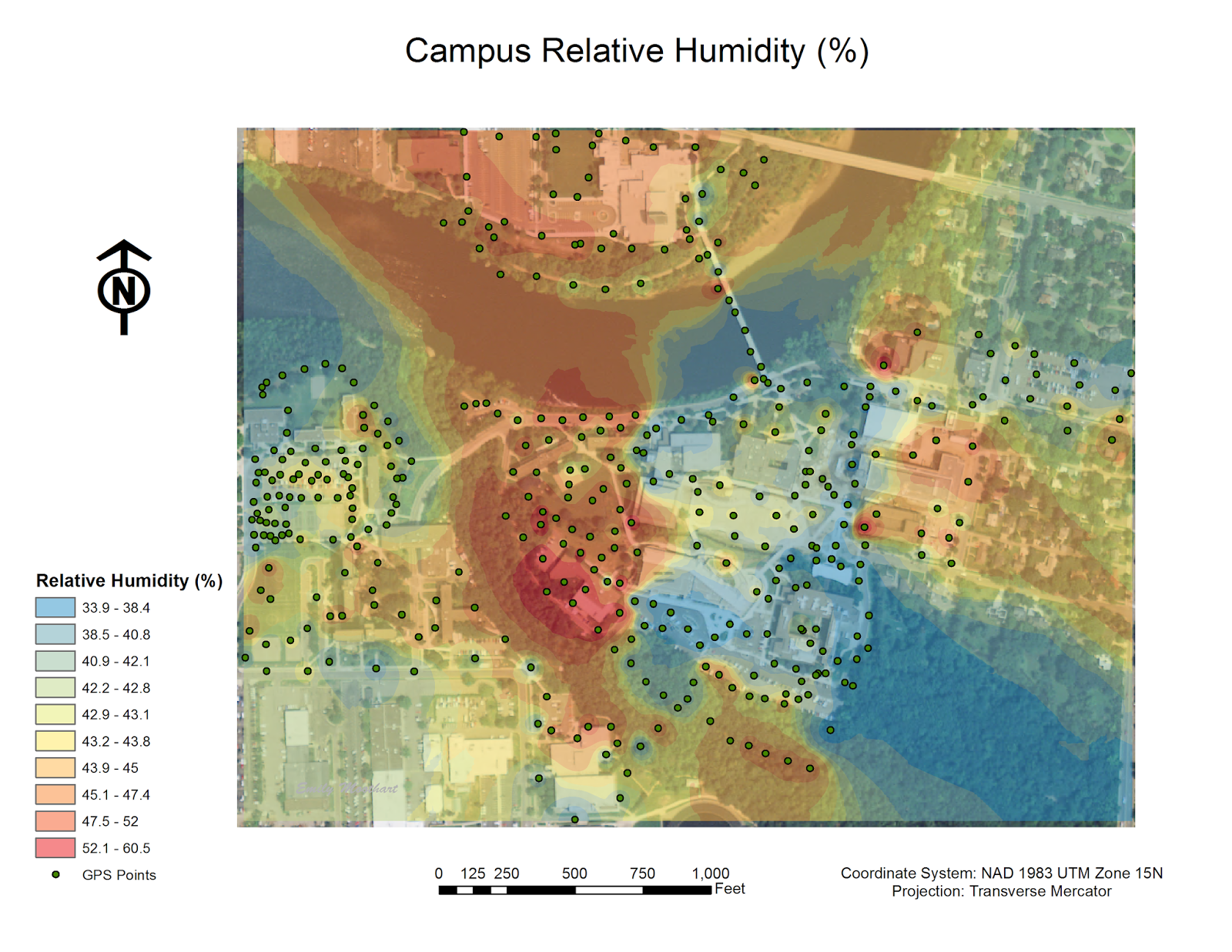 |
Figure 5. Campus Relative Humidity. This map is almost identical to the dew point map above. The regions that have higher humidity are generally areas around open water, or in the case of this particular day on campus, waterlogged because of the snow melting. The lowest relative humidity is located in the general area of the parking lots which contain a lot of cement, and likewise almost no water. |
Temperature is an easy to understand concept that really aids in the initial understanding of a microclimate (see figure 6). Although at first it did not make sense that the yellow symbol was consuming the map, it was realized that the range of the symbology was between 0 and 60 degrees Fahrenheit. This is obviously an input error that effected the output of the map display.
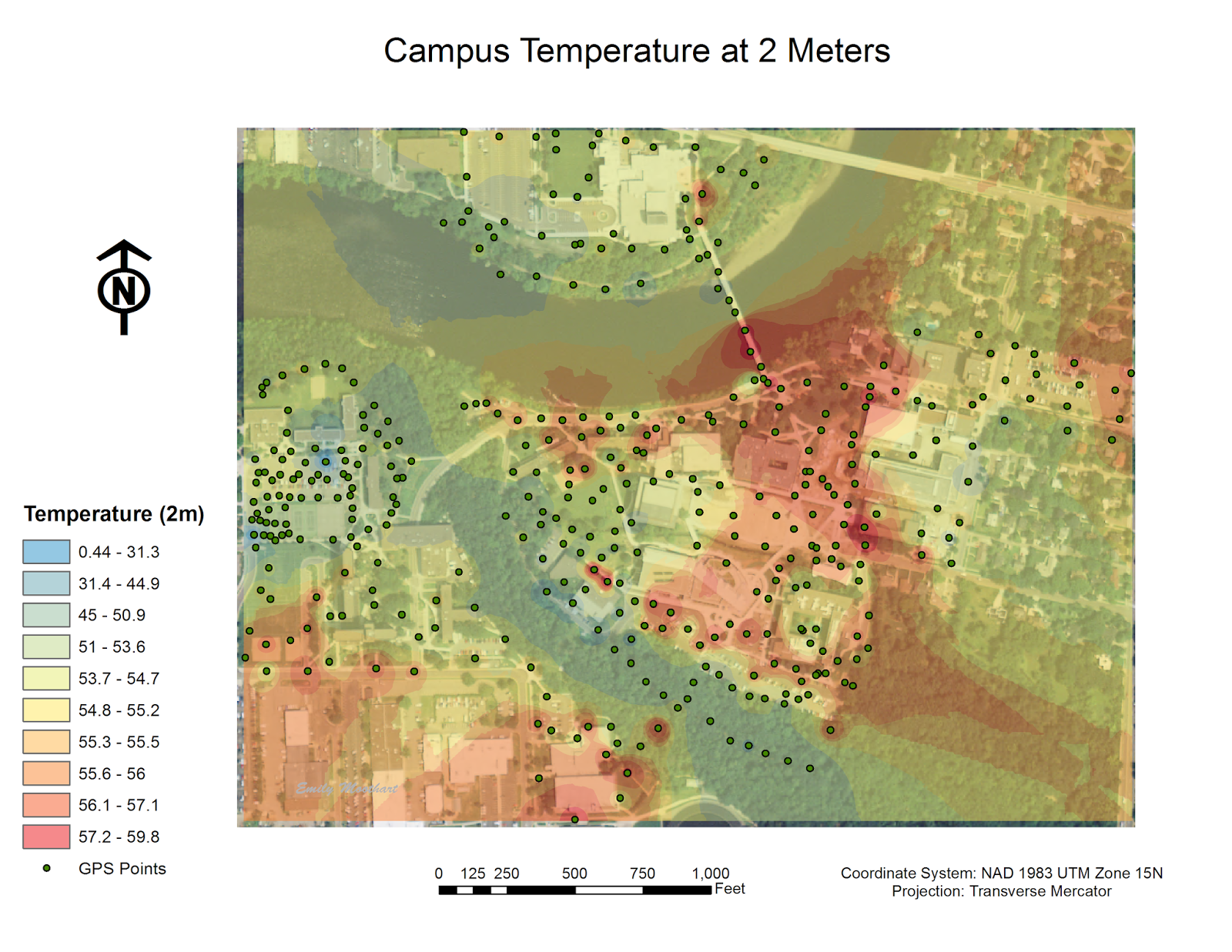 |
Figure 6. Campus Temperature at 2 Meters (Fahrenheit). The coldest regions on the map are the ones located under tree cover. After analyzing the attribute table, it was realized that one of the groups neglected to include any two meter data. The 'null' area is shown in the middle of the red segment in the east-central portion of the map. With more data, there would have likely been more variation in interpolation output. |
Although temperature at the surface should be very similar to the temperature at two meters, it is still important to collect the data to compare the variation between the two temperature readings (see figure 7).
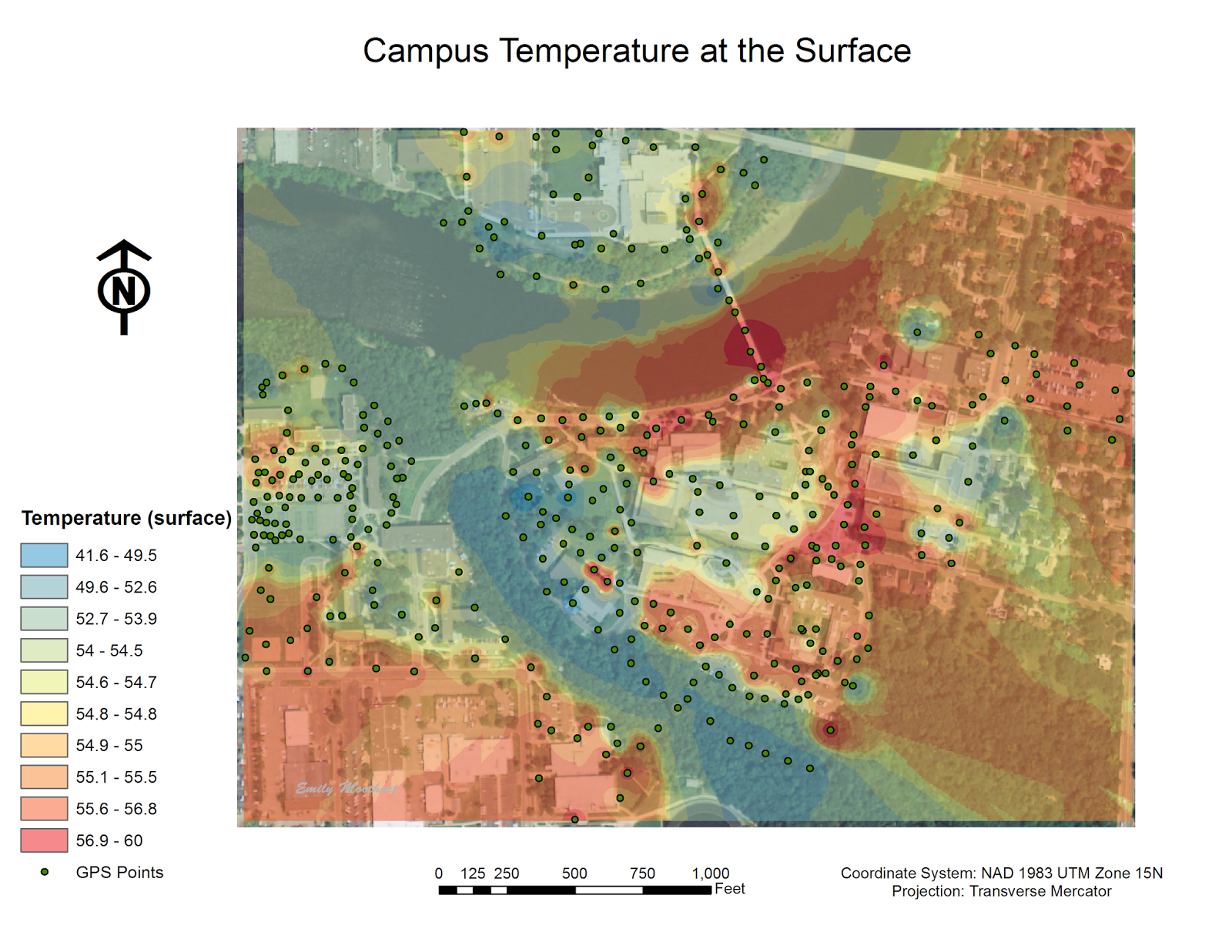 |
Figure 7. Campus Temperature at the Surface (Fahrenheit). The coldest regions on the map are the ones located under tree cover. This is similar to the campus temperature at two meters. |
Relatively high wind speed have an effect on this campus largely due to the Chippewa River running through it. Below is a map indicating the wind speeds, in miles per hour, throughout the campus (see figure 8).
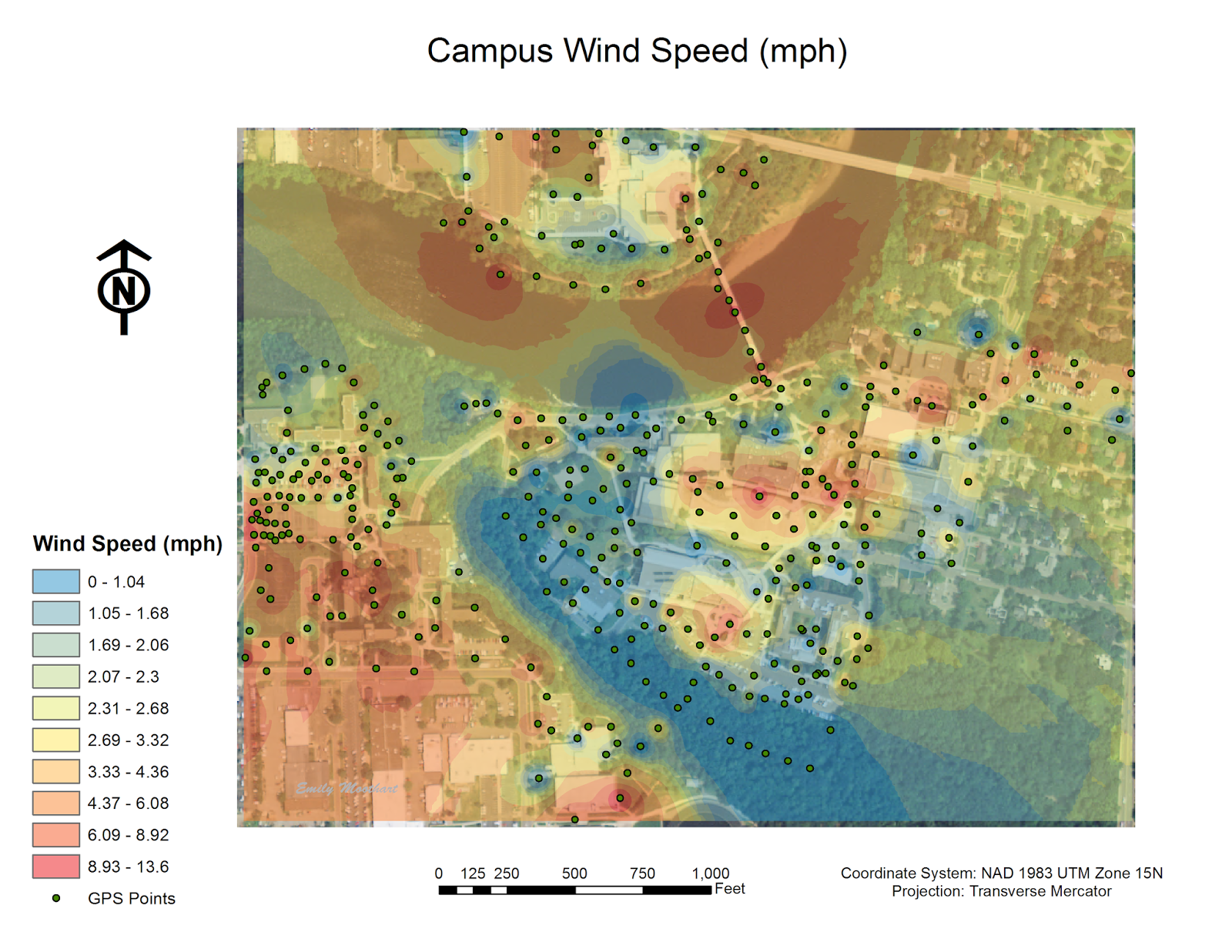 |
Figure 8. Campus Wind Speed (miles per hour). There is a direct correlation to where the tree cover is and the wind speed. In Putnam part, there were no wind where there is the most tree cover. In addition, exposed areas along the river's shore have higher wind speeds. |
Wind chill is another weather variable that was measured (see figure 9). Wind chill is the perceived decrease in air temperature felt by the body on exposed skin due to the flow of air. Wind chill is always lower than the air temperature when the formula is valid. The issue with using the Kestrel is that there are often readings of the wind chill that are higher than the temperature of the surface or at two meters, which is inaccurate.
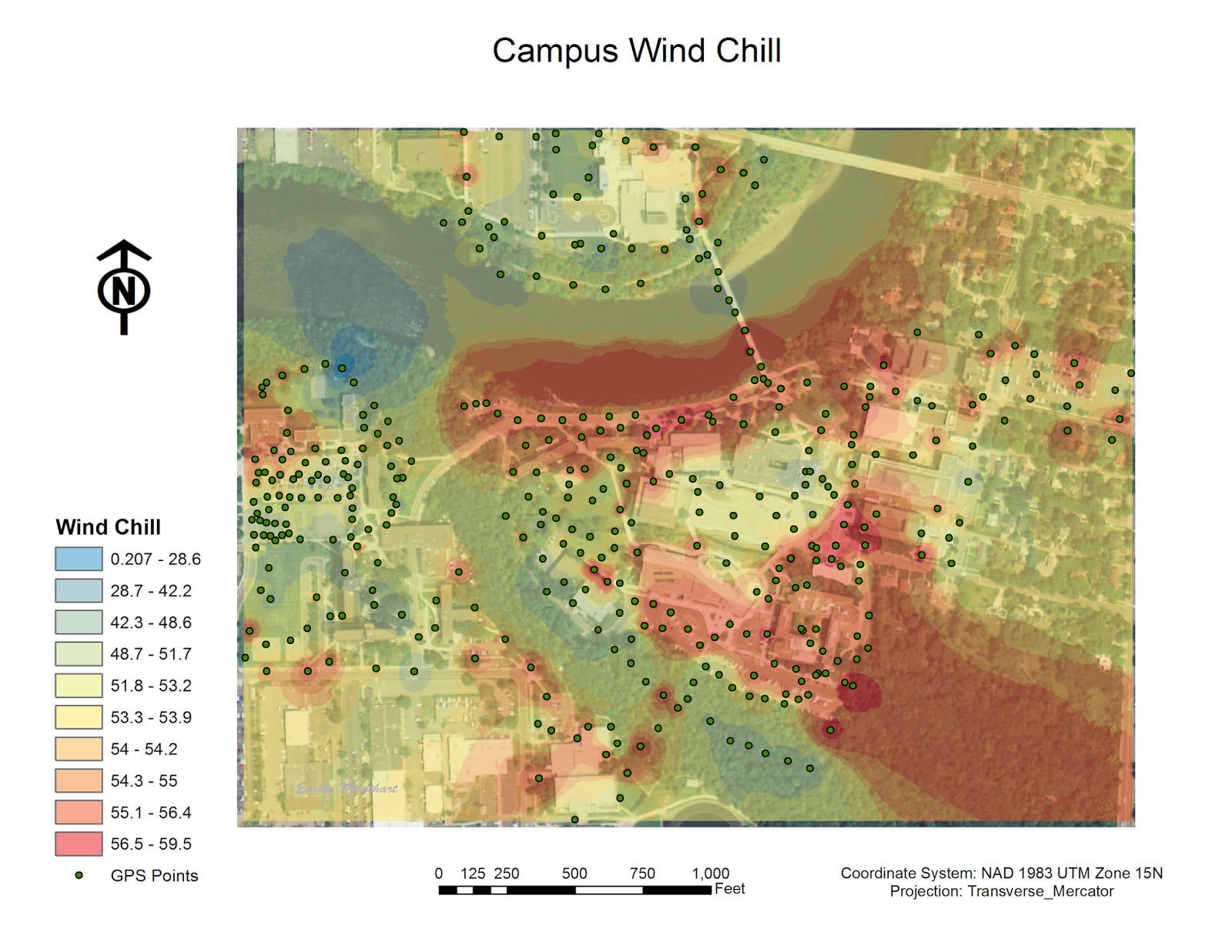 |
Figure 9. Campus Wind Chill (Fahrenheit). Not surprisingly, the highest wind chill was along the river. It was surprising when analyzing the data that there was still fairly strong winds in parking lot behind the Davies Student Center (the lower central area indicated in red). |
Ground cover is determined as the terrain type on a given surface such as grass, black top, snow, gravel, open water, sand, concrete, and other (such as woodchips). There were a couple of points in the data set that were missing the ground cover data, so those few points were determined as 'undefined' on the map below (see figure 10). Ground cover plays a potentially important role in the measurement of certain weather conditions. One of the more important measurements that would be variable due to this is temperature at two meters and temperature at the surface. If the day had been quite sunny the black top would have likely been much warmer at the surface than at two meters, which would have affected the outcome. According to the data collection records, there really is not much of a difference between the two temperature readings, so this factor does not seem to have played a role on the microclimate data taken on this particular day.
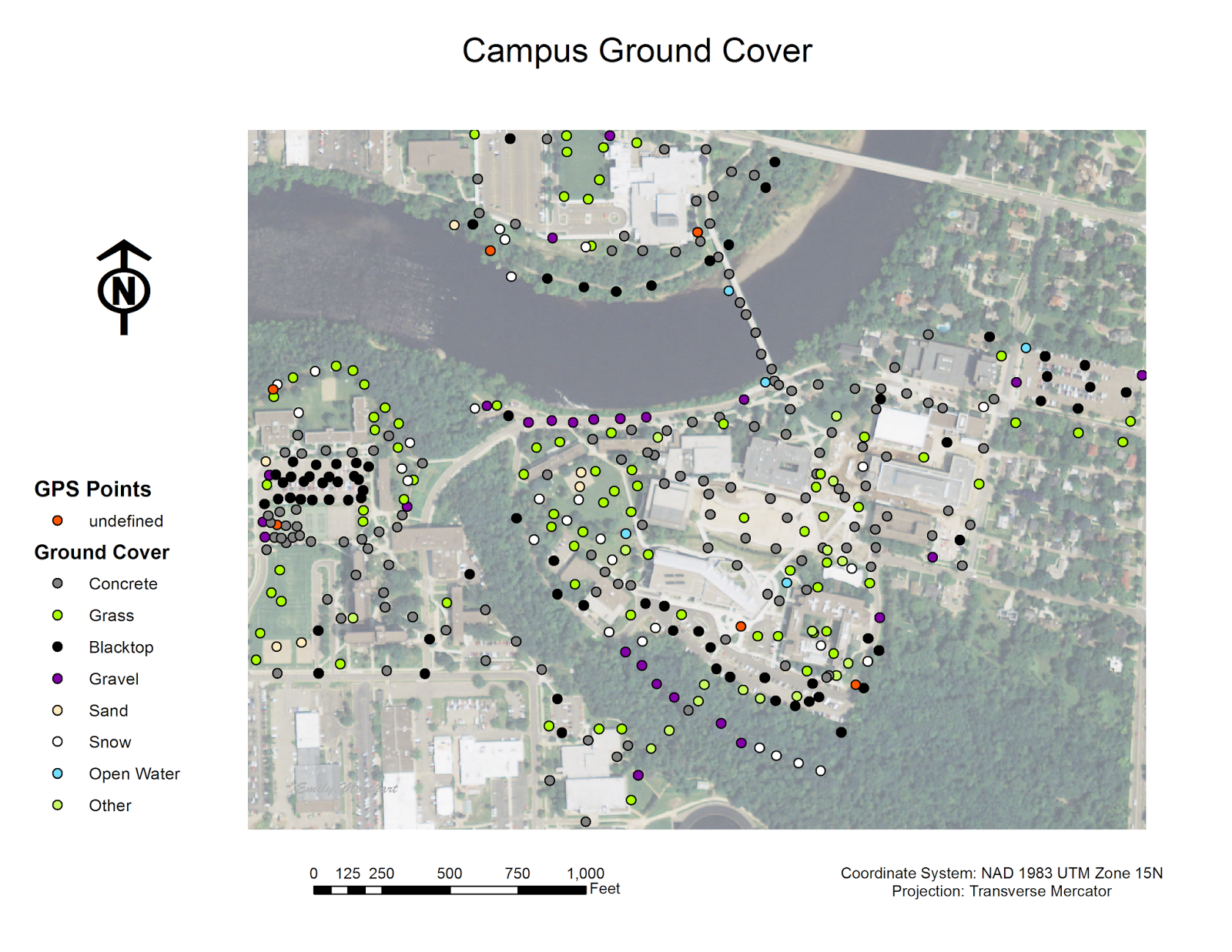 |
Figure 10. Campus Ground Cover. This displays the variation in surface features in which the data was collected on. |
Discussion
From the last geospatial data collection, the temperature outside increased about 30 degrees Fahrenheit. This shows that even if you believe the domain originally set will cover the likely temperatures for the time of year, think again. It is definitely better to have a larger sampling of GPS points; comparatively to last week's test run, this grouping of points allows for a much more detailed spatial analysis.
However, by looking in the attribute table for the data points, there were many errors when inputting the data. One error is that a group failed to measure the temperature at two meters for all of their designated region (see figure 11). Additionally, another group entered '0's into their temperature at two meters column (see figure 12). Both of these mistakes would therefore impact the visual outcome of the IDW interpolation that was performed.
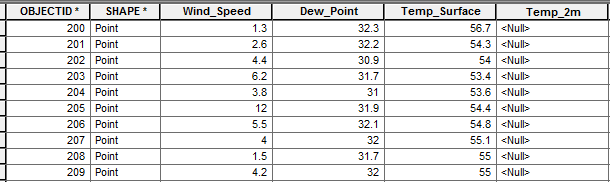 |
Figure 11. As seen in the 'Temp_2m' column, there were no recorded measurements at two meters, which would have affected the interpolation output for the map. |
 |
Figure 12. An error was made in the data input of 'Temp_2m' in that '0' was entered, which is a clear outlier in the data. This would therefore skew the interpolation output and range for the data as a whole. |
Regarding the data collected, I was surprised that there was not more of a distinctive microclimate impression directly from the presence of the Chippewa River. There were regions along the river that indicted a similar microclimate, but I would have thought that it would have been more consistent along the river's shore.
In addition, after the three years of living on campus, I have realized that climbing up the stairs to get to the top of the hill next to the river generally has a drastic change in temperature and wind speed as one walks up. It would be intriguing next time the survey is done to take a point at every set of stairs to see the a possible change in climate.
Although we collected all of the points within a two hour period, there was still a lot of variability when it came to the weather readings, especially wind speed in a given minute. To me, this makes it very difficult to overall gather an accurate reading of the campus microclimate. In addition, the class failed to effectively use the notes field during the data collection out in the field. It would have been interesting to know other weather conditions that could have played a part in the outcome of the data.
Conclusion
This particular lab ran quite smoothly because of the attention to detail in the steps prior to the data collection. There would have been a much different discussion if we had been inefficient in deploying our database or if there had been a failure in the database. Due to human error, there are a few circumstances in which the data is incorrect, but as a whole, the microclimate lab was a success. That simply shows that one needs to take time when doing all portions of the lab, and that missing one step can effect the entire class's interpretation of the data.
I feel like after this lab I have a really good handle on how to create a reliable geodatabase and know how to confidently deploy it, collect the data, bring it into ArcMap, and analyze the data. This knowledge will help immensely in future geographical activities so that more attention can be given to the data analysis, not just the data collection. Having this course has really helped with gaining a solid understanding of how to use ArcMap in many applications that will be useful in the future.